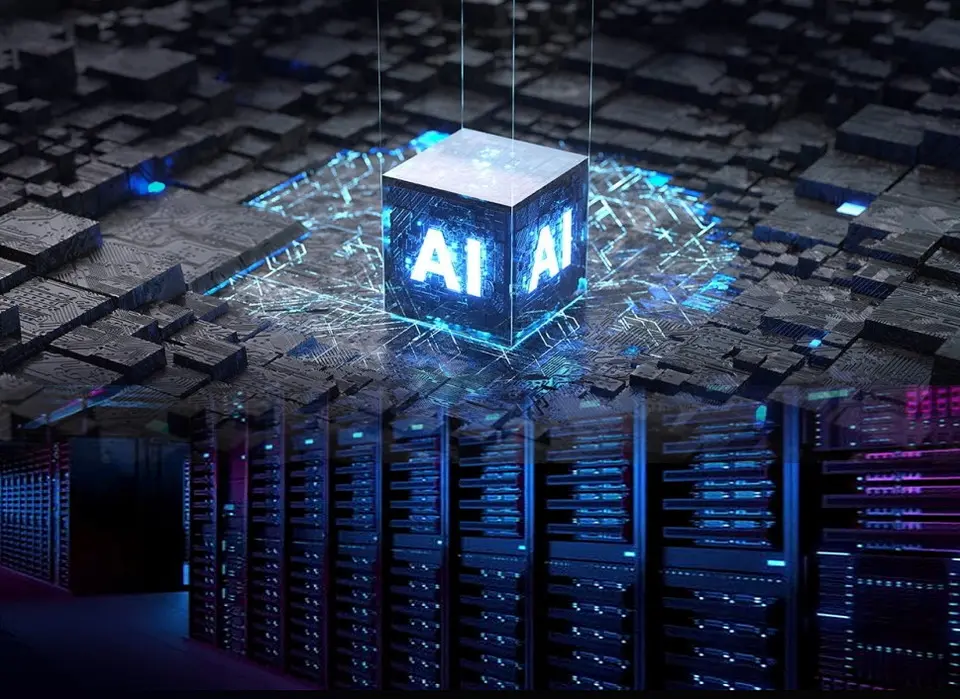
In modern society, data has become a more crucial resource than ever before. As the importance of time-varying data continues to grow, the need for tools to effectively manage and analyze this data is emerging. Against this backdrop, Time Series Databases (TSDB) are gaining attention, and their value is further increasing through integration with Artificial Intelligence (AI) technology. In this article, we will take an in-depth look at the synergy between TSDB and AI.
Time Series Databases and AI
A time series database is optimized for storing and managing data that is measured at regular intervals along a time axis or recorded whenever events occur. Examples of time series data include stock price fluctuations, daily temperature changes, and changes in a patient's heart rate. The characteristics of these TSDBs are gaining more attention in conjunction with recent AI developments. This is because AI has an inseparable relationship with data learning and analysis, and it is recognized as one of the few viable solutions for efficiently processing the explosively increasing amount of data.
Benefits of AI Utilization through TSDB
First, there is the efficiency of AI training data management. The performance of AI systems is largely dependent on the quantity and quality of data. TSDB provides the ability to efficiently store and manage large-scale time series data, allowing quick access and processing of the vast amounts of data needed for AI model training. In the case of recent large language models (LLMs), the amount of data to be trained has increased by tens to hundreds of times compared to existing methods, requiring management of data volumes that are difficult to handle with the simple expression "there's a lot of data". For example, even when collecting general vibration data, one month's worth of data can amount to over 70 billion records, and in situations where this needs to be extracted and inferred in real-time, the role of TSDB becomes even more important.
Second, there is an increase in AI learning accuracy. One of the main areas where AI is applied to time series data is in various prediction models through pattern analysis. Examples include predicting stock market price fluctuations, weather forecasts, and machine failure predictions. To increase the precision and accuracy of these prediction models, accurate and consistent data is essential. TSDB's large-scale data management technology contributes to improving data quality, resulting in improved performance of prediction models and enabling more accurate predictions. In particular, maintaining and learning various types of data becomes a key factor in determining the quality and performance of AI, so the accuracy of TSDB and AI models can be seen as having a perfect causal relationship.
Third, there is improved accuracy in anomaly detection. Another important area where AI is applied to time series data is anomaly detection. Anomaly detection here doesn't simply mean sounding an alarm when a problem occurs, but detecting anomalous signs before a problem occurs. While this is a form of the prediction model mentioned earlier, it's worth addressing separately due to its specificity. For anomaly detection, it's first necessary to define the range of normal patterns, which requires learning from a large amount of data. TSDB is not only suitable for processing large amounts of data, but it can also quickly store and query real-time data, enabling real-time anomaly detection. This plays a particularly important role in situations requiring immediate response, such as detecting abnormal traffic patterns in network security.
TSDB and LLM Technology Integration
With the recent advancement of AI technology, new ways of combining with TSDB are gaining attention. One of these is the fusion of large language models (LLMs) and TSDB. LLMs are models trained on large-scale text data and show excellent performance in the field of natural language processing (NLP). When LLMs are applied to time series data, they can understand patterns in time series data and explain them in text format, or conversely, convert text data into time series format. For example, LLMs can understand users' natural language queries and generate responses in natural language based on time series data stored in TSDB. They can also be used to write automated reports such as "Weekly Weather Reports" or to explain the results of complex prediction models in an easy-to-understand way.
However, new methodologies are needed to effectively apply LLMs to TSDB. One that has been gaining attention recently in this regard is RAG (Retrieval-Augmented Generation). RAG is a methodology that uses external information to enhance the text generation capabilities of LLMs, searching (Retrieval) for relevant information from external databases or documents before generating information, and then generating (Generation) responses based on that information. The format in which LLMs are applied to TSDB follows this RAG form. Specifically, the LLM understands the user's natural language query and converts it into a query suitable for searching data in the TSDB. Then, RAG retrieves relevant time series data from the TSDB, and based on the retrieved data, the LLM generates a response in natural language. In this way, the combination of LLM and TSDB is realized through RAG, combining language models and information retrieval technology to generate more accurate and relevant responses.
In the future, time series databases are expected to play an even more important role in the development of AI. Through the encounter with new technologies such as LLM and RAG, they will be more closely integrated with AI, enabling AI models to learn time series data in real-time and provide prediction results in real-time. Furthermore, prediction models will continuously learn new data and automatically update to constantly improve prediction accuracy.
In particular, the fusion with LLM and RAG is expected to contribute to building more advanced AI systems by combining natural language processing and time series data analysis. This will play an important role in greatly enhancing the user experience utilizing time series data and increasing business value. For example, it will be possible to provide complex time series data analysis results in a form that even general users can easily understand, or to gain insights into time series data by asking questions in natural language.
In the Future
In conclusion, along with AI, time series databases will play an increasingly important role in a data-centric world. The synergy between these two technologies has the potential to not only increase the efficiency of data management and analysis but also fundamentally change the way we understand and predict the world. This is expected to lead to innovation in various fields and ultimately contribute greatly to improving the quality of human life. We now stand on the threshold of infinite possibilities that the perfect union of time series databases and AI will bring. It is noteworthy where this exciting journey will lead us.